123Fab #12
1 topic, 2 key figures, 3 startups to draw inspiration from
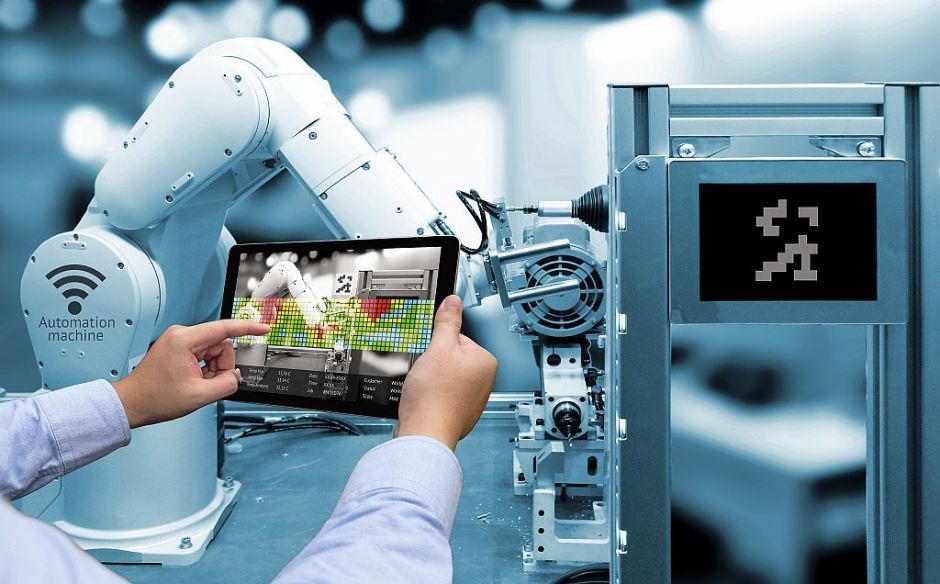
Smart Maintenance Operations: from preventive maintenance to predictive maintenance
According to a study conducted jointly by KPMG and L’Usine Nouvelle, two-thirds of the companies surveyed consider the increasing availability of equipment in factories to be a top priority over cost reduction and quality improvement. In fact, plant downtime can be very costly — ranging from $10,000 to $50,000 per minute — depending on the industry (e.g. $22,000 for the automotive industry). However, wrench time in factories has remained stable for almost a decade, demonstrating a real gap between the need for productivity and maintenance efficiency.
In the past, maintenance services were mainly corrective and preventive, meaning that equipment was repaired in the event of an immediate failure or default, or that preventive maintenance operations were scheduled based on time and usage parameters. This maintenance management was based solely on the assumption that the equipment would be degraded after a certain period of time.
Over the past two decades, the adoption of digital technologies has paved the way for smart maintenance. In particular, advances in data management have made it possible to shift from preventive to condition-based maintenance. Condition-based maintenance monitors the condition of an asset in real-time to determine when a parameter reaches an unsatisfactory level in order to plan a maintenance intervention.
More recently, the ongoing transformation of manufacturing practices, driven by machine learning, artificial intelligence and automation, has led to the rise of Smart Maintenance in factories. Several startups are now positioning themselves on this market and developing innovative solutions to optimize and facilitate maintenance operations, based on 3 main pillars:
- Asset monitoring and geolocation, which uses data analysis to provide real-time visibility on the location and condition of the materials and assets within the supply chain. As part of asset monitoring, predictive maintenance allows to accurately predict when a component will fail and determine when to repair it, reducing downtime costs. It often involves the creation of a Digital Twin, a digital replica of a physical asset, developed to replicate the original behavior model.
- Reverse engineering and 3D scanning technologies, which uses data to create 3D virtual models for Computer Aided Manufacturing and Computer Aided Design softwares. These models can be used as a complement to virtual and augmented reality to train workers to maintenance operations.
- Blue Collar Empowering technologies, such as voice recognition, instruction digitalization and project management softwares, which improve worker performance. These technologies are efficient assistants because they save maintenance workers from typing their maintenance or reading complex manuals.
In conclusion, Smart Maintenance has become crucial to maximize uptime and reduce production costs, but also to extend equipment lifetime, ensure compliance and enhance security and energy consumption. However, manufacturing companies may be a little reluctant given the significant investment involved in the transition to Smart Maintenance, especially in this time of crisis. Smart maintenance operations also addresses the critical need for energy optimisation. In France, 20% of energy savings in the industrial sector can still be easily achieved through smarter equipment. Aster Fab is committed to the energy transition in the industrial sector through the INVEEST Programme, an initiative that supports financial and industrial professionals in their transformation. Please contact us to learn more.
2 Key Figures
135 Digital Twin startups
are focusing on developing solutions for the Logistics, Supply Chain, and Maintenance sectors
Market size expected to reach $12.3bn by 2025
According to Markets & Markets, the global predictive maintenance market was valued at $4bn in 2019 and is expected to reach $12.3bn by 2025.
3 startups to draw inspiration from
This week, we identified three startups that we can draw inspiration from: Falkonry, Polyga, and Datch Systems.
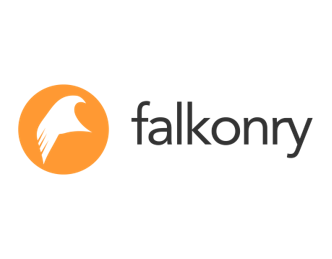
Falkonry
Falkonry is a US-based startup offering a ready-to-use machine learning system that helps companies improve their industrial operations in terms of performance, throughput, quality and yield.
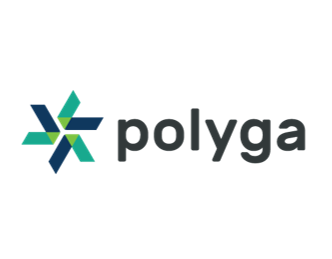
Polyga
Polyga is a Canadian startup developing 3D scanners and a mesh processing software to facilitate the use of scan data within Computer Aided Design and Computer Aided Manufacturing systems.
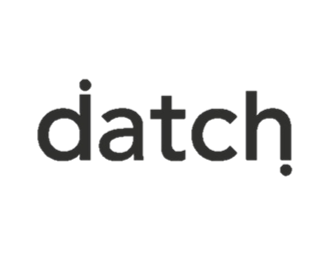
Datch Systems
Based in the UK, Datch Systems develops an intelligent voice interface for industry, enabling blue workers to use their voice to document their work.