123Fab #65
1 topic, 2 key figures, 3 startups to draw inspiration from
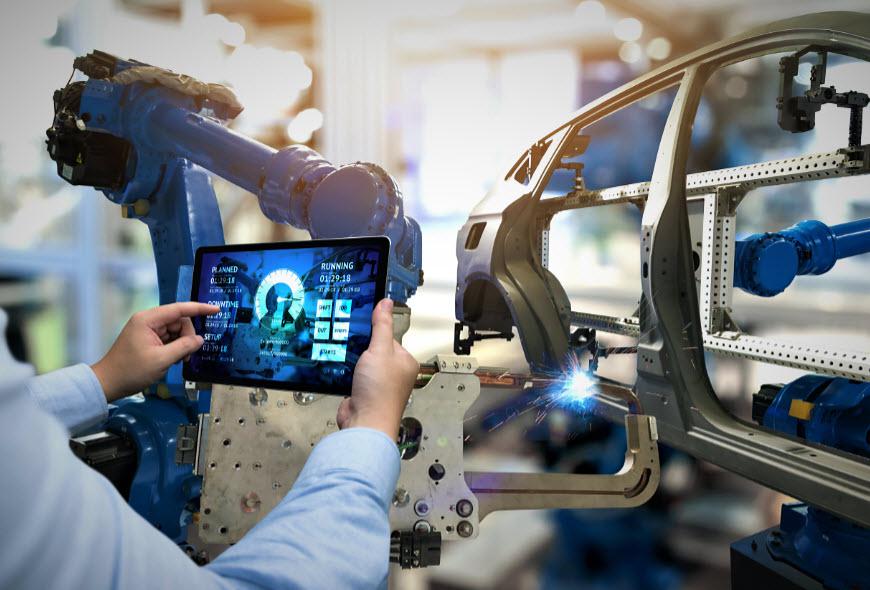
In March 2020, the European Commission presented an industrial policy to support the twin green and digital transitions to make the EU industry more competitive globally, and strengthen Europe’s open strategic autonomy. Indeed, today’s industry, and more particularly the manufacturing sector, has a real problem of productivity and difficulties to innovate, especially in comparison with other sectors. As an illustration, the manufacturing sector in the US has experienced the steepest decline in productivity growth of any sector, according to Economics TD. In the EU, productivity growth for industrial companies fell from an average of 2.9% over the 1996–2005 period to just 1.6% percent from 2006–2015. This can be explained by the fact that digitalization in manufacturing is low compared to sectors such as transportation, utilities or finance. Manufacturing also represents less than 10% of venture capital investments in the US and EU. This looks set to change, as Industrial Tech Investment levels have grown 8.8x since 2014, nearly three times faster than overall European VC investment. AI-based use-cases can really help improve the productivity of manufacturing companies.
AI can improve the productivity of the manufacturing sector at different levels. First, it can help to optimize processes. Machines become self-optimized systems that adjust their parameters in real-time by continuously analyzing and learning from current and historical data. It can also be used for predictive maintenance by continuously analyzing machine data to predict and avoid breakdowns. AI may help to automate quality control by using image or time-series sensor data to identify defects and deviations in product features. Finally, AI can be used to train cobots and enable them to perform a wide range of tasks.
Some large companies have started to integrate AI into their operations to improve productivity. For example, Mitsubishi Electric has developed its own system internally to adjust the parameters of its industrial robots. It has reduced process times by 90%. But overall, according to PWC, only about 9% of manufacturing companies have successfully implemented AI in their operations. They face certain difficulties, particularly in the development and deployment of AI models. Factory data can come from many sources and in many forms, making data collection and integration challenging. Labeling manufacturing data is also a cumbersome and time-consuming process. It often requires domain knowledge which means that manufacturing companies may be reluctant to work with labeling service providers. There are also many challenges in terms of security, processing power, scalability and explainability.
This is why startups seem to have a role to play alongside manufacturers. Startups can help in a variety of activities: improve data sourcing, improve data labelling and quality, facilitate model deployment and provide final use cases. Some players have already joined forces with startups such as Alcoa, which partnered with the UK startup Senseye to build a predictive maintenance solution. In total, Alcoa has reduced unplanned downtime by nearly 20%. A French Tier-1 automotive manufacturer partnered with the startup Scortex to automate the inspection of painted plastic parts. Scortex’s solution reduced inspection time by 80%. There is also a growing appetite among venture capital firms to invest in this sector. Cognite, which provides an IIoT platform as well as use-cases such as predictive maintenance, raised €128m in May 2021, Kili Technology which enables large companies to transform their raw data into high-quality annotated data raised €21m in June 2021…
However, the market is young, small and niche, which explains the low number of startups that manage to emerge. For data sourcing, hyperscalers like Amazon or Microsoft or incumbents like Siemens are competing with startups, and in-house solutions are being developed by industrial companies. With the exception of time-series data labeling and quality monitoring, there is no need for a specific tool for manufacturing and a generalist tool can be applied (H2O.ai for example is an AI Cloud Platform designed to operate in any environment).
In short, it is clear that AI and more specifically machine learning are full of promise for the manufacturing sector, to improve processes and boost productivity. However, the market is still not yet mature and developed. Startups attempting to conquer the market seem to have difficulty scaling or to competing with hyperscalers like Amazon. As a result, generalist tools are being applied to the manufacturing sector, especially in the deployment of models. It is likely that such tools will see a stronger adoption from the manufacturing sector as it matures, but the first step is to improve data sourcing, annotation and quality monitoring.
2 Key Figures
The Global Artificial Intelligence in Manufacturing Market is expected to reach $11.5 Bn by 2027, with a growing CAGR of 27.2%
The market was valued at $2.1bn in 2020 – AllTheResearch (Sept 2021)
318 funded companies in AI in manufacturing
$3.1bn total funding and $1.5bn funding in last 2 years – Tracxn (Sept 2021)
3 startups to draw inspiration from
This week, we identified three startups that we can draw inspiration from: Scortex, Senseye and Kili technology.
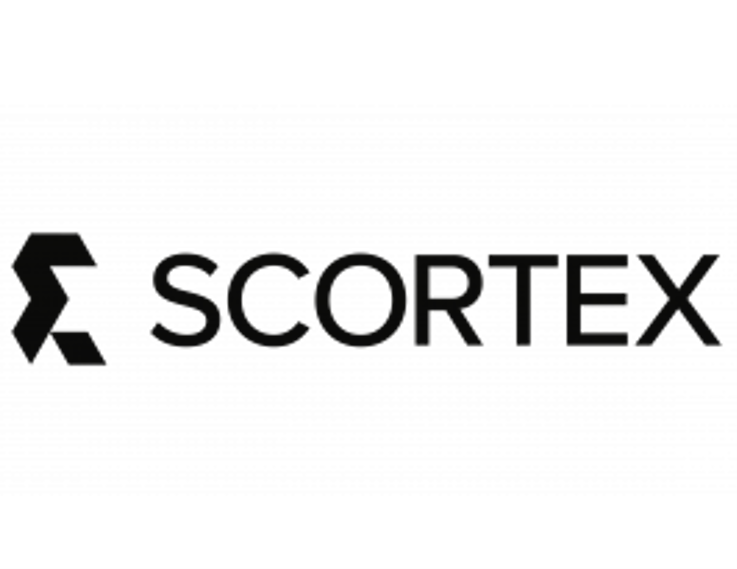
Scortex
The startup has developed a a machine intelligence platform designed to set up a solution for the factory production line. It transforms quality inspection and offers an automated defect detection and analytics solution to more accurately identify defective products in real-time.
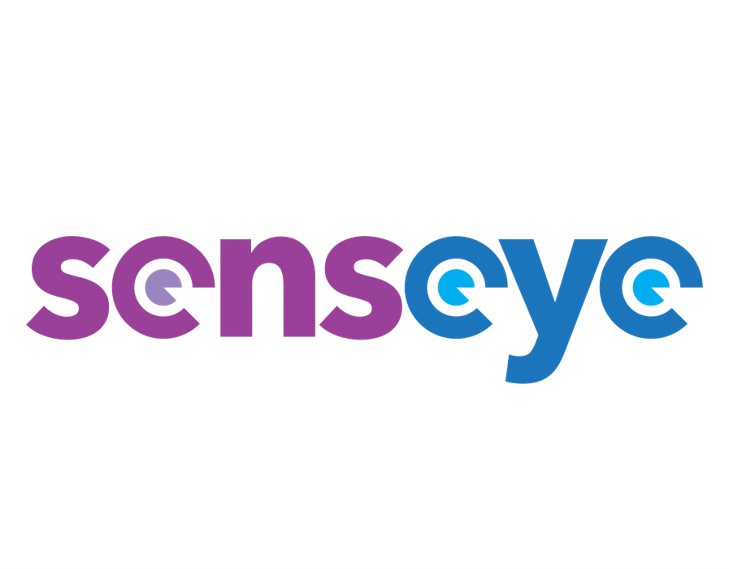
Senseye
The startup has developed a cloud-based machine monitoring and diagnostics platform intended to deliver a way to predict machine failure. The platform automatically tracks and sends depreciation data of machinery and notifies manufacturers about machine conditions.
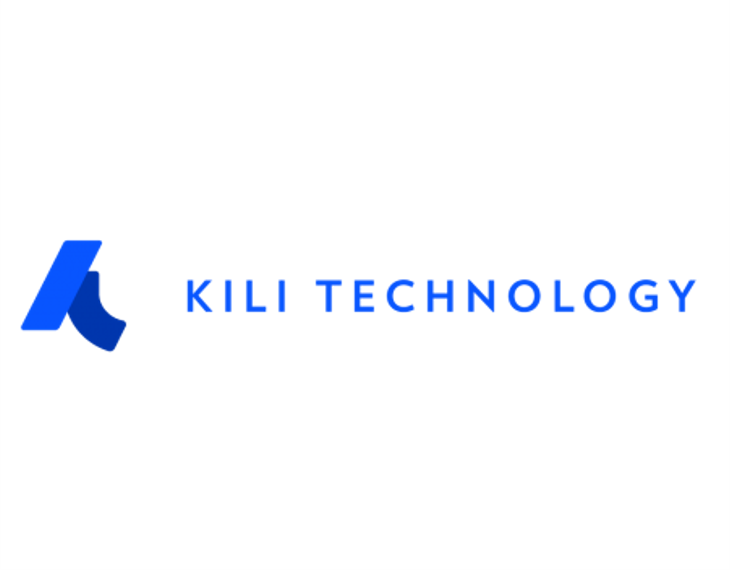
Kili technology
The startup has developed an annotation platform intended to create and manage data sets for artificial intelligence training. The platform allows for easy management of the training data for annotation, quality control, data management and labeling workforce.
Interested in a startup landscape or in an insights report?
Please fill out our contact form so that we can get back to you very quickly with our product offer.
Want to subscribe to our 123Fab?
Fill out our form to receive the latest insights into your inbox.