123Fab #51
1 topic, 2 key figures, 3 startups to draw inspiration from
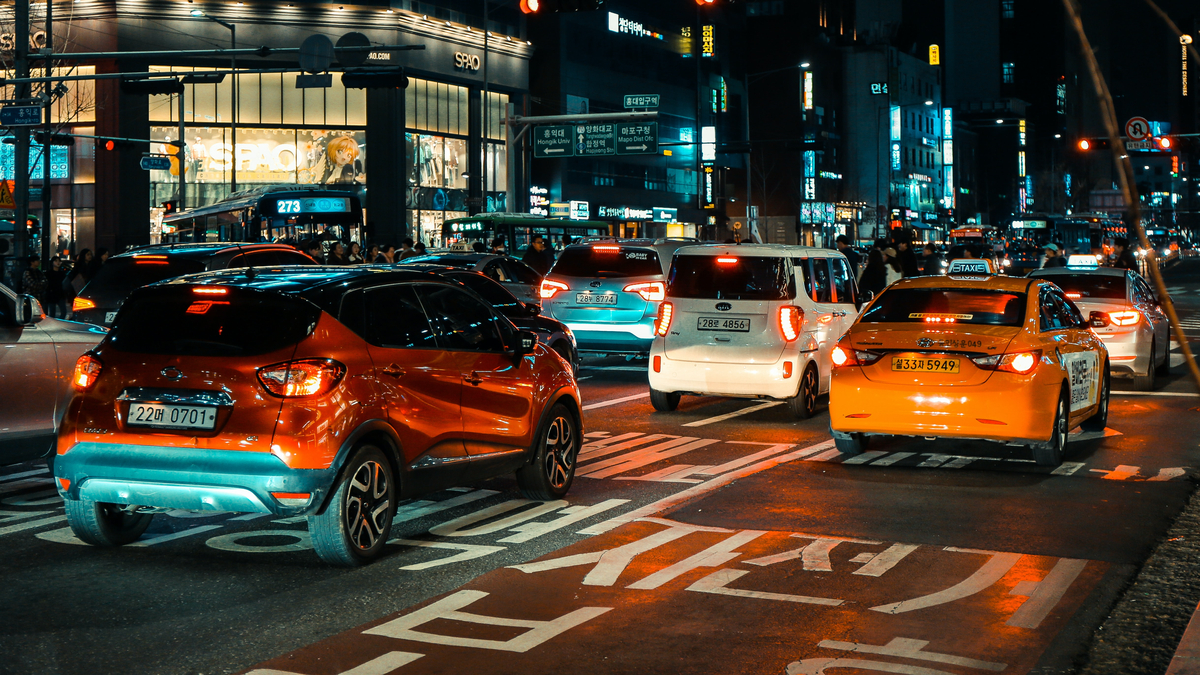
Behind the concept of the autonomous car lies 6 levels of automation ranging from 0 (classic car) to 5 (fully autonomous). Today, most commercially available vehicles are at most level 2: partial automation. At level 3, the vehicle can take full control of driving in certain situations (such as in traffic jams) — these vehicles are just coming to market.
Over the past decade, there has been much hope that automated cars would arrive in the near future, but now this prospect seems more remote, with the sale of Uber’s self-driving department 6 months ago not boding well for the industry in the short term. So what went wrong? What are the issues that the carmakers’ $120Bn investment over two years could not solve?
Part of the answer lies in the myriad of potential situations one may encounter while driving. Autonomous cars work well in controlled environments, but to achieve level 4 or 5 autonomy, i.e. letting go of the steering wheel for most of the journey, they need to be fully prepared for any situation (e.g. snow covering road markings, areas with no coverage, street lighting malfunctioning, jaywalking pedestrians, etc.). Thus, training artificial intelligence is essential for its public adoption, which is what OEMs, startups and Tier-1s are doing.
Training of algorithms for the multiplicity of situations
One step towards fully autonomous driving is to assess the safety of the AI driving model and highlight its blind spots to improve training on these cases. Startups such as Phantasma Labs have developed a virtual testing environment to assess the model’s behavior when confronted with Vulnerable Road Users (VRUs) such as pedestrians or cyclists. The model is faced with millions of situations involving machine learning-based VRUs in order to study the driving and address the mishandled situations. Other unexpected objects can disrupt driving. If traffic signs, stands, barriers, or excavators on the road seem straightforward to avoid for a human driver, it is more difficult for AI that has never encountered it before. Deep Safety offers annotated datasets dedicated to training models for the construction or road work industry. Its own trained AI identifies unknown objects encountered, raising an alert for the driver to take over. To evaluate the general safety of a model’s driving skills, Ivex proposes a safety assessment tool that provides KPIs on the safety of trajectories taken by the autonomous driving system, either in simulation or on real driving data, to spot potential improvements.
Drawing on as much data as possible
It seems unlikely that traditional cars will disappear in the near future and the behaviour of human drivers is harder to predict than that of driverless cars. Startups are also addressing the subject by connecting traditional and driverless cars. Valerann builds road sensors that gather information for autonomous vehicles about their in-lane location, even when the markings are not visible. They also connect classic cars to driverless vehicles by sharing their exact location and predicted trajectory, spotting abnormal driving behavior, and warning surrounding cars of danger. Eyenet offers collision prediction software based on GPS data from phones and AI that could be used to connect regular vehicles to autonomous ones and detect and prevent potential collisions. All this information is very valuable. The more accurate real-time data the model has, the easier it is to make the right decision.
React as quickly as possible
Training more complex models, fed by more information and capable of handling a wider range of situations, comes at a price. In the case of autonomous vehicles, every millisecond counts in the decision-making process, which puts great pressure on the data transmission process as well as on the computational power that can be installed in the car. Regarding data transmission, 5G and smart cities will have a great role to play, and the technology is on its way. When it comes to enhancing the car’s computational performance, edge computing seems to be the most promising answer. Unlike cloud computing, where calculations are performed on a remote server, far from the data, edge computing solutions offer calculations in microdata centers, relatively close to the car. This proximity tackles two problems: firstly, for autonomous cars, lag would have disastrous consequences and the lagging risk in communication is reduced when the data is sent to a closer location. Secondly, privacy is increased and the hacking risk is reduced as the data arrives in a smaller, more controlled environment than the cloud. Vapor.io provides microdata centers designed for edge computing that could be used for autonomous vehicles. Quadric.io is developing edge computing processors optimized for real-time calculation that will allow deeper models to be run. AlphaIcs has also developed its Real AI Processor (RAP), an edge computing solution designed for heavy AI applications like real-time automated driving. Together, these solutions address the trade-off between computing power and network latency for autonomous cars.
Startups appear to be leading the way in fully autonomous car innovation, thanks to massive funding from OEMs: Toyota and Aurora announced a partnership 4 months ago to produce mass autonomous vehicles for ride-hailing networks like Uber. Last year, Jaguar Land Rover partnered with Waymo to develop Waymo’s driverless fleet, and Volkswagen invested $1 billion in Argo’s self-driving technology and its Autonomous Intelligent Driving department became part of Argo.
It is difficult to assess when, or even if, autonomous vehicles will be the most represented on the road of the future, but applications are definitely emerging be it for ridesharing, trucking, or deliveries, market leaders are investing and technology is going forward.
2 Key Figures
129 self-driving car startups
registered by Tracxn
Global autonomous car market expected to reach $1,642 Bn by 2025
The global autonomous car market was estimated at $818.6 Bn in 2019 and is expected to reach $1,642 Bn by 2025, at a CAGR of 17.4%
3 startups to draw inspiration from
This week, we identified three startups that we can draw inspiration from: Phantasma Labs, Quadric.io and Eyenet.
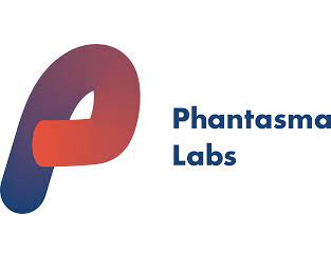
Phantasma Labs
Founded in 2018, Phantasma Labs is a Berlin based startup that developed artificial intelligence-based massive simulations designed to improve self-driving automobiles. The company’s platform generates a substantial amount of data-sets related to real-life situations at scale for self-driving cars, models the behavior of pedestrians, cyclists and other road users in large-scale simulations in virtual scenarios, enabling autonomous vehicle makers to learn from real-life situations without the consequences of accidents.
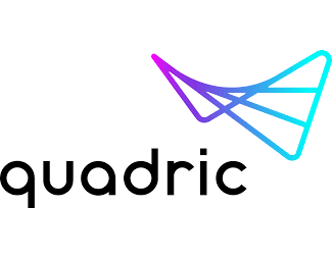
Quadric.io
Based in California, Quadic.io developed an edge processor designed to meet the needs of next-generation autonomous products, Industrial IoT products, and robots. The company’s processors can be incorporated into a wide range of products that require instantaneous processing of real-world data streams with minimal power and maximum speed as well as commute safely, industrial robots to complete critical tasks and create human interaction with machines, enabling developers to empower with tools to create tomorrow’s technology today.
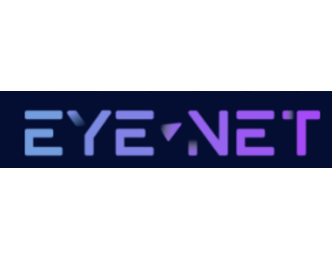
Eyenet
Created in 2018, Eyenet developed a collision prediction and prevention software-based platform designed to addresses safety challenges in the shared mobility landscape. The company’s platform incorporates AI-powered algorithms that calculates user location and collision probability as well as utilizes a sophisticated probability analysis for spatial cross-correlation of bearing, velocity and acceleration to determine an imminent collision.